
Subscribe & Follow
The power to know: How data science is transforming retail
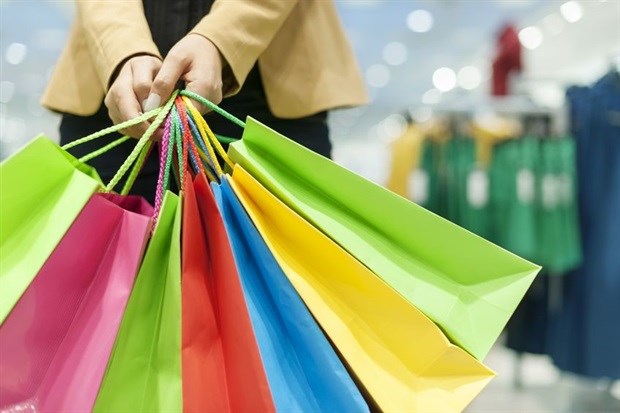
To succeed in the new digital retail environment, companies must learn how to collect and then use the raw data generated by the technology that underpins everything. Combined with advanced techniques and technologies, the base metal of data is transmuted into digital gold: actionable insights and business value where it matters most - the bottom line.
The necessity for data science in retail is widely recognised, with most operators appreciating that being competitive depends on consistently delivering great customer experiences. Retailers comprehend that if their competitors are using data science to understand customers better, and then offer them what they want, they will have to use the same tools to better effect.
That much is apparent from the 2017 Zebra Retail Vision study, which showed that retailers are planning investments in the Internet of Things (70%), machine learning and cognitive computing (68%) and automation (57%).
But first, let’s consider where raw data in retail comes from.
Rich in raw data
It should come as no surprise that retailers have always generated plenty of their own data, both in-store and throughout the supply chain. However, historically speaking, for most retailers, somewhere between very little and nothing has been done with that data.
This is changing, driven in part by the big online retailers like Amazon and AliExpress, which have introduced new and exciting customer experiences that rest on data science. In particular, Amazon’s pioneering work in data science can truly be said to have disrupted the entire retail sector.
Today, with the inexorable march of technology, the amount of data generated by all retailers has increased dramatically. Furthermore, there are additional data stores available which can enhance the “raw material” available for analysis.
Examples of the traditional data sources include product and pricing information, customer purchase, credit and payment history, and various other transactional data. Newer data stores which can be “ingested into a data processing platform include external customer data from social media platforms or government sources like the Census, real-world data like weather and holiday dates and, increasingly, sensor data including that generated by Internet of Things deployments linking millions of inanimate objects to the network.
Turning data into information
But data is just data. It isn’t the same thing as information – and that’s where data science comes into it, along with techniques like machine learning, artificial intelligence and predictive modelling.
Data science combines human capabilities and mathematics with specialised software tools to process and analyse data. For retailers, three overlapping possibilities emerge from data science:
1. You can increase revenues and retain customers with accurate personalised recommendations Amazon-style. Data analysis can segment customers, so you can focus on the most profitable ones, while understanding key metrics like their likelihood of making purchases and lifetime value analysis.
2. You can optimise stock holding and distribution to reduce working capital and warehouse space. Analytics provides insights including more accurate demand forecasting, optimised routing and scheduling for an efficient supply chain, and optimised picking and packing for a high-performance warehouse.
3. You can maximise credit profits and minimise losses. With accurate insights, you’ll know with a high degree of confidence which customers are worthy of credit, and which are potentially risky. You’re also equipped to manage churn by being able to identify customers who are likely to stray and put in place retention strategies. Analytics even provides insights to optimise collections for customers who haven’t paid their bills.
If that all sounds a little too theoretical, it is useful to break down data science into a series of questions to which every retailer is likely to relate. These questions can be grouped in terms of:
• Product: Are you carrying customer-centric localised assortments (the right goods at the right time)? What is behind sale and margin fluctuations? What is the current stock situation and is an out-of-stock likely?
• Pricing: What are the pricing sweet spots? How do price adjustments affect sell-through? What tactics can be employed to manage markdowns and control margin erosion?
• Promotions: What are the best next-sell; upsell and cross-sell opportunities? What products do customers typically buy together? Can promotion spend be optimised?
• Product placement: Are products meeting sales targets? What impact can repositioning have on specific items? What is the yield effectiveness of shelf, aisle and end-cap placements?
• In-store: Are stores staffed and managed optimally? Do store associates have real-time customer insights? Are customers provided with personalised experiences?
The possibilities offered by data science for retailers are nothing short of transformative. By combining data generated automatically every day in the business of retail with those additional data sets from social media, the supply chain or elsewhere, data science helps you understand your customers far more intimately. By converting raw data into information that is relevant to the retailer, data science gives you the ability to optimise your operations from one end to the other.
This retail-specific information provides one of management’s most potent tools: the essential metrics that signal success, and thus the power to measure how well you are doing.
In conclusion, it should also be emphasised that in order to take full advantage of the insights offered by data science, profound organisational and cultural shifts are necessary. Working with the right partner to effect this mix of technology and behavioural change can make all the difference in getting this complex transformation right.
About Marko Salic
Marko Salic is CEO of the Argility Technology Group, an innovative software development group of companies with a 30 year plus history originating in the retail and financial sectors. Marko has over 20 year's industry experience, stemming from software development and architecture through to business development, management and strategy. He spent 15 of those in Argility creating some of the company's most innovative solutions and products.